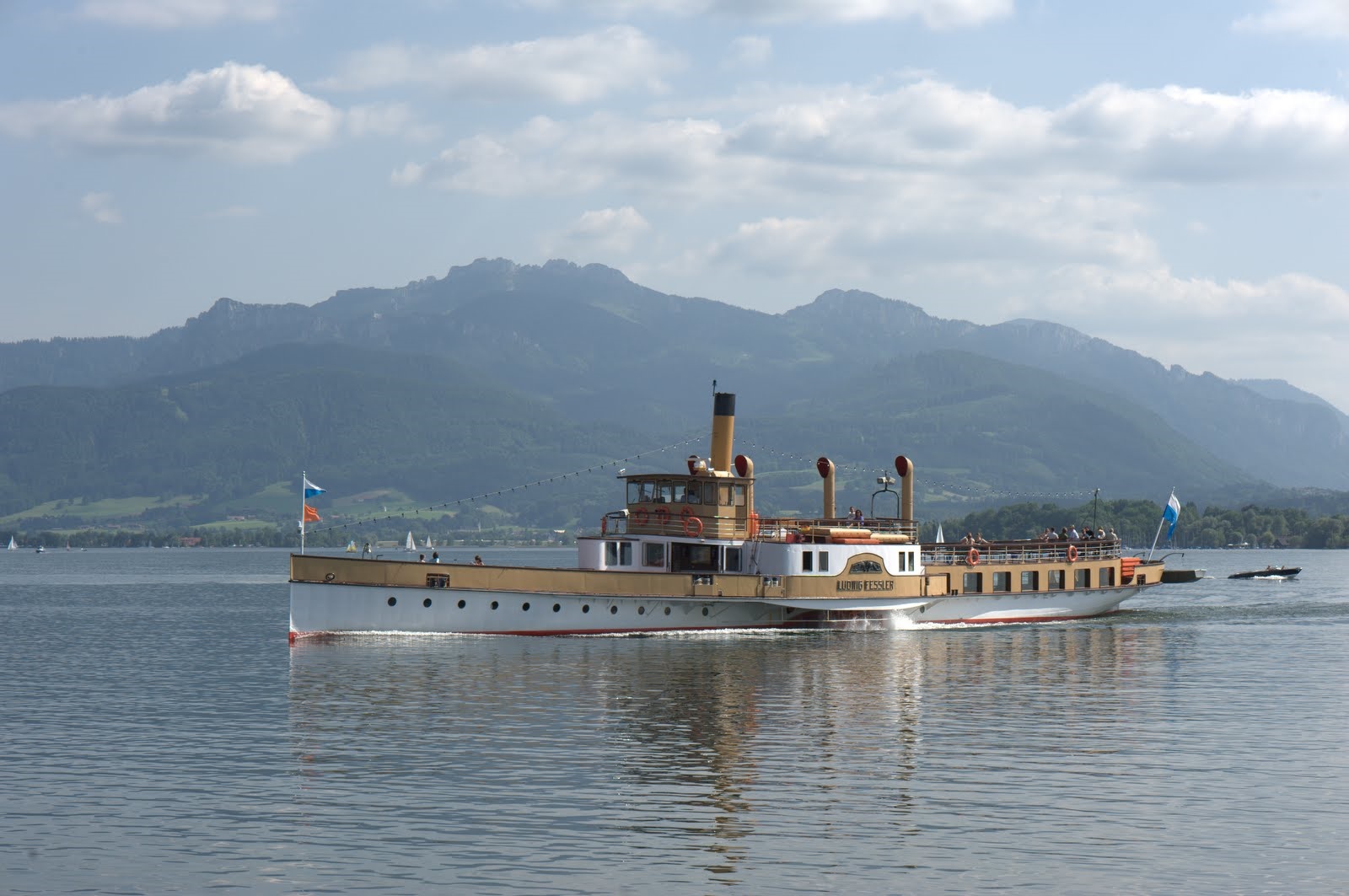
- Opettaja
Alexandra Virtanen
The seminar is intended to be a roundtable for computer science (CS)
graduate students and researchers. It is based on contemporary academic
and professional materials in and related to CS.
The course introduces methods and algorithms for extracting information and knowledge from data sets. This includes techniques for data pre-processing, visualizing high-dimensional data, basic machine learning methods for supervised learning (classification, regression), unsupervised learning (clustering, association rule analysis), model selection and validating how well a learned model predicts on new data (holdout, cross-validation). The CRISP-DM process model is introduced as a tool for analysing and implementing data science projects.
Prerequisites: Python programming skills. Basic knowledge of probability, statistics and linear algebra is beneficial. Taking the course TKO_7093 Statistical Data Analysis before this course is recommended.